21st International Conference on Computational Methods
©LMIH/Focalize
Invited Speakers
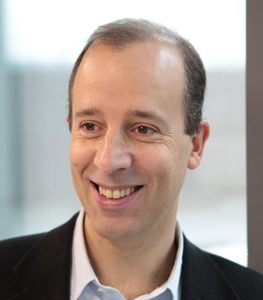
Jorge Goncalves (University of Luxembourg, Luxembourg)
Title: Causal dynamical network inference: from theory to applications in biology
Abstract:
One of the major objectives in systems biology is to extract information from data that can be used to make reliable predictions. In most cases, however, this is very difficult due to the limited amount of data. One major obstacle is the lack of mathematical tools tailored to the specific needs of systems biology. This talk presents both theoretical and practical advancements in building dynamical network models from limited time-series data. The theoretical aspect discusses experimental requirements that guarantee identifiability of dynamical networks. The application part illustrates recent tools developed in our group applied to a wide range of experimental data, including bulk and single cell data.
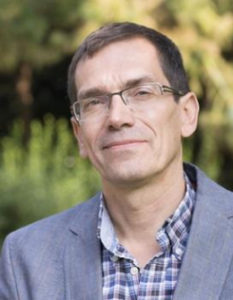
Philippe Jacques (University of Liège, Belgium)
Title: Optimizing NonRibosomal Peptide Synthesis by Biocomputing Approaches
Abstract:
The biosynthesis mechanism of NonRibosomal Peptides (NRPs) was elucidated for the first time in 1965 with the description of the NonRibosomal Peptide Synthetase (NRPS) of the gramicidin from B. subtilis. As suggested by their name, these peptides are not assembled on ribosomes but by large multienzymatic proteins that are encoded by gene clusters that could span over tens of kilobase in the genome. More than 500 different monomers can be incorporated in such peptides leading to a high structural biodiversity of NRPs with various interesting biological activities (antimicrobials, immunomodulators, biosurfactants,…) and a high set of applications in different sectors (pharmaceutical, agronomical, chemical, environmental…).
Improving the biosynthesis of NRPs is thus frequently an important objective that can be managed by rational metabolic engineering approaches such as: (i) blocking competitive pathways for building blocks, as well as, those pathways that consume products; (ii) pulling flux through biosynthetic pathways by removing regulatory signals; and (iii) by overexpressing rate-limiting enzymes.
One way to develop this metabolic engineering approach is to use knockout of genes which negatively influence the intracellular pool of monomer precursors. To do that, the precursor metabolic pathways have to be modeled as a reaction network taking into account the regulation processes. The choice of those knockouts can be directed by methods from computational biology, to narrow them down and reduce the laboratory time needed. Some prediction methods are related to formal reasoning techniques based on abstract-interpretation of reaction networks with partial kinetic information. While being a general framework for the static analysis of programming languages, abstract interpretation is applied here to steady state equation of the reaction network, in order to enable qualitative reasoning with logical methods. On example of this approach will be developed for the overproduction of a NonRibosomal lipopeptide produced by Bacillus strains, the surfactin. It shows strong biosurfactant activities and is able to stimulate plant defense mechanisms.
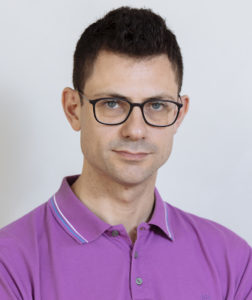
Mirco Tribastone (IMT School for Advanced Studies Lucca, Italy)
Title: Lumping and Expanding Reaction Networks
Abstract:
Reaction networks are a fundamental tool to model systems across many branches of science. Still, our ability to gain mechanistic insight and effectively conduct numerical evaluations is critically hindered when dealing with large models. We will discuss a family of reduction algorithms for reaction networks that yield a self-consistent system through a linear projection of the original state variables. In addition to speeding up computation times, these approaches enable the physical interpretability of the aggregation in applications to computational biology, evolutionary game theory, and network science. We will also investigate the opposite problem, namely the expansion of reaction networks to improve their accuracy when they represent first-order approximations of continuous-time Markov chains, especially under bursty behavior, multi-scale separation, and multi-stability conditions.
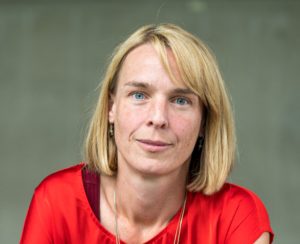
Verena Wolf (Saarland University, Germany)
Title: Integrating Machine Learning and Mechanistic Modeling: Unlocking the Potential of Hybrid Approaches in the Life Sciences
Abstract:
In the life sciences, both machine learning and mechanistic modeling are widely employed methodologies. Machine learning offers data-driven solutions for regression and classification problems, while mechanistic modeling focuses on describing real-world systems and validating hypotheses about them.
In this presentation, I will explore the strengths and limitations of machine learning and mechanistic modeling, highlighting instances from the life sciences where a hybrid approach combining these two paradigms has successfully enhanced our understanding of the underlying systems. Furthermore, I will discuss various methods for incorporating mechanistic relationship information into machine learning models, particularly neural networks, and demonstrate the immense potential of these hybrid models.
Important dates
- Abstract of regular papers submission: 23 April 2023 (extended)
- Paper submission: 30 April 2023 (extended)
- Notification: 9 June 2023
- Camera-ready: 30 June 2023 (extended)
- Poster/Highlight talk: 9 July 2023
- Conference: 13-15 September 2023
Proceedings published in:
Sponsored by:
- Department of Computer Science, University of Luxembourg
- Springer Nature